The trade finance gap continues to impact businesses around the world, but is the problem technical or procedural, and can AI offer an effective response? We ask an expert.
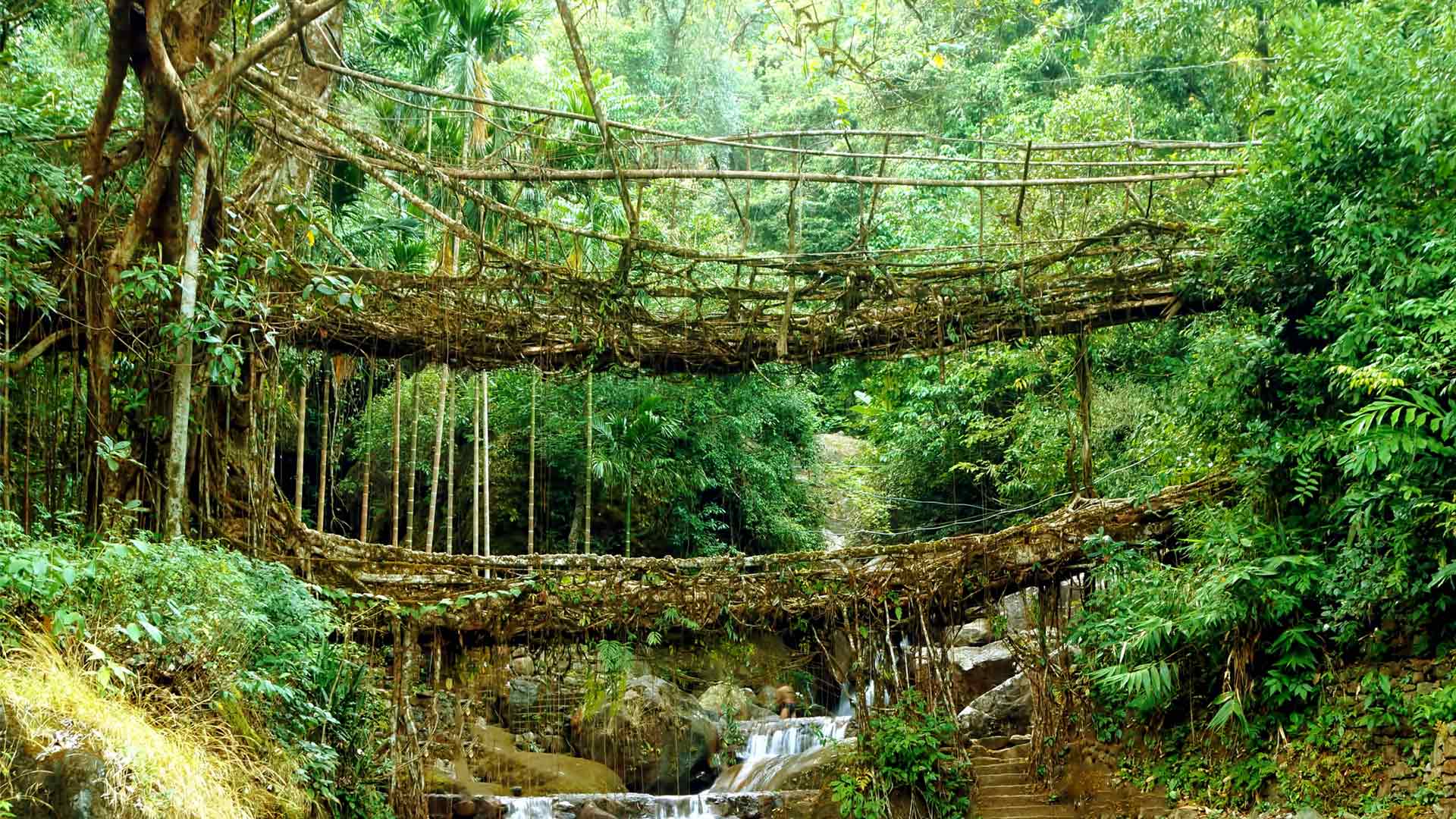
When new rules are made, unintended consequences often arise; that’s just how it is. The 2008 financial crisis lead to stringent know your customer (KYC) checks, which made it harder for businesses in the developing world to supply the KYC information in the required format. Where banks are unable to appropriately assess the risk in a transaction, they will price accordingly. This is a particular issue for small businesses and those in emerging economies who often don’t have the financial history or data to be effectively ‘KYC’d’.
The unintended consequence, notes Michael Walker, Head of Working Capital Finance, Asia Pacific, Finastra, has directly resulted in a trade financing gap of US$1.5trn for SMEs globally, and 45% of financing requests from SMEs being rejected.
The COVID-19 pandemic has exacerbated this situation and the current financing gap is likely to rise sharply as a result. The World Trade Organisation forecasts global trade volumes will drop by up to 32% and the International Chamber of Commerce (ICC) predicts US$5trn of additional trade credit will be required to bring trade back to pre-pandemic levels.
Procedural problem
“Yet the problems with trade finance are also procedural,” says Walker. Indeed, according to the ICC, a single trade finance transaction can require over 100 pages of documents, and there are an estimated four billion pages of physical, paper documents in circulation at any one time. The same data points are repeated across multiple documents, and these documents are mostly physical originals when it comes to global trade and trade finance.
“When a letter of credit is used to mitigate risk of non-payment between buyers and suppliers, consistency is critical not only across the pages in the document set, but also between the document set and the letter of credit they are presented under,” he says. It can take up to two days and considerable expertise to check a set of documents, and mistakes can be costly; not only in terms of the fees charged by banks but also from the delay in being able to clear goods at the end destination. “It is not uncommon for goods to arrive at their destination before documents clear the banking system, adding additional warehousing, storage and demurrage charges.”
The high cost and risk of delay in traditional trade finance processing has contributed to the rise of Open Account financing, which accounts for up to 80% of global trade. Though Open Account financing is riskier as a trusted intermediary and is not used to mitigate risk of non-payment, it is more cost-effective and efficient. The challenge for banks with Open Account trade, says Walker, is that they typically only have visibility of the payment between counterparties, making anti-money laundering checking process more difficult and more expensive, impacting on the trade finance gap.
AI role
“Artificial intelligence (AI) and applied machine learning, in parallel with more widespread digitalisation of trade processes, documents and data, can help to close the trade finance gap and reduce the impact of COVID-19 on global trade,” Walker believes. “Through being able to make more effective use of data that lies unstructured in physical trade documents, and add context by connecting different data sources, a more complete picture of a trading party can be obtained.”
Certainly, increased visibility reduces risk in transactions and allows corporates and banks to secure supply chains by going deeper with financing. The emergence of distributed ledger technology (of which blockchain is a type of), and it’s inherent characteristics like data immutability and smart contract support, further reduces the need for data duplication and supports process automation.
“In order to prepare for AI adoption, corporates and their treasuries need a ‘digital first’ mentality,” Walker advises. This means embracing process automation and cloud-based solutions for dynamic management, integration and on-going analysis of datasets.
“They should look at how open APIs and platform-based business models can be leveraged to enable them to seamlessly integrate data from third party sources, giving them greater visibility and risk mitigation capabilities when looking to on-board new counterparties and build resilience into their supply chains.”
Corporates should also look to rationalise their IT infrastructure, he advises. “They may be connecting disparate systems through bespoke interfacing today, or using legacy processes, host-to-host connections or even using spreadsheets. Corporates need to connect these internal data sources and add context through external data sources to support their adoption of AI.”
Data exchange
From a bank’s perspective, faster, more predictable and repeatable analysis of datasets, and the ability to connect data sources from inside and outside of their organisation, will allow them to build up a better picture of their corporate client and its business. Through analysis and monitoring of cash flows and accurate forecasting of financing requirements, banks can dynamically manage limits and optimise their capital allocation.
Furthermore, a deeper understanding of their corporate client “opens up the possibility of more effective targeting of value-add services, while AI based transaction repair instead of traditionally inflexible rules-based approaches can also be supported”, says Walker.
Moreover, increasing the level of automation in processing enables banking operations or corporate treasury personnel to manage by exception or focus on value-add tasks. “Together, these factors contribute to a reduction in transaction risk and allow banks to lower their cost of financing, helping to lower a current barrier to entry for the SME and MSME sector.”