Artificial intelligence and machine learning are becoming commonplace in the business world, but there are numerous factors that corporates need to consider before they rely on this technology and do away with the need for humans altogether.
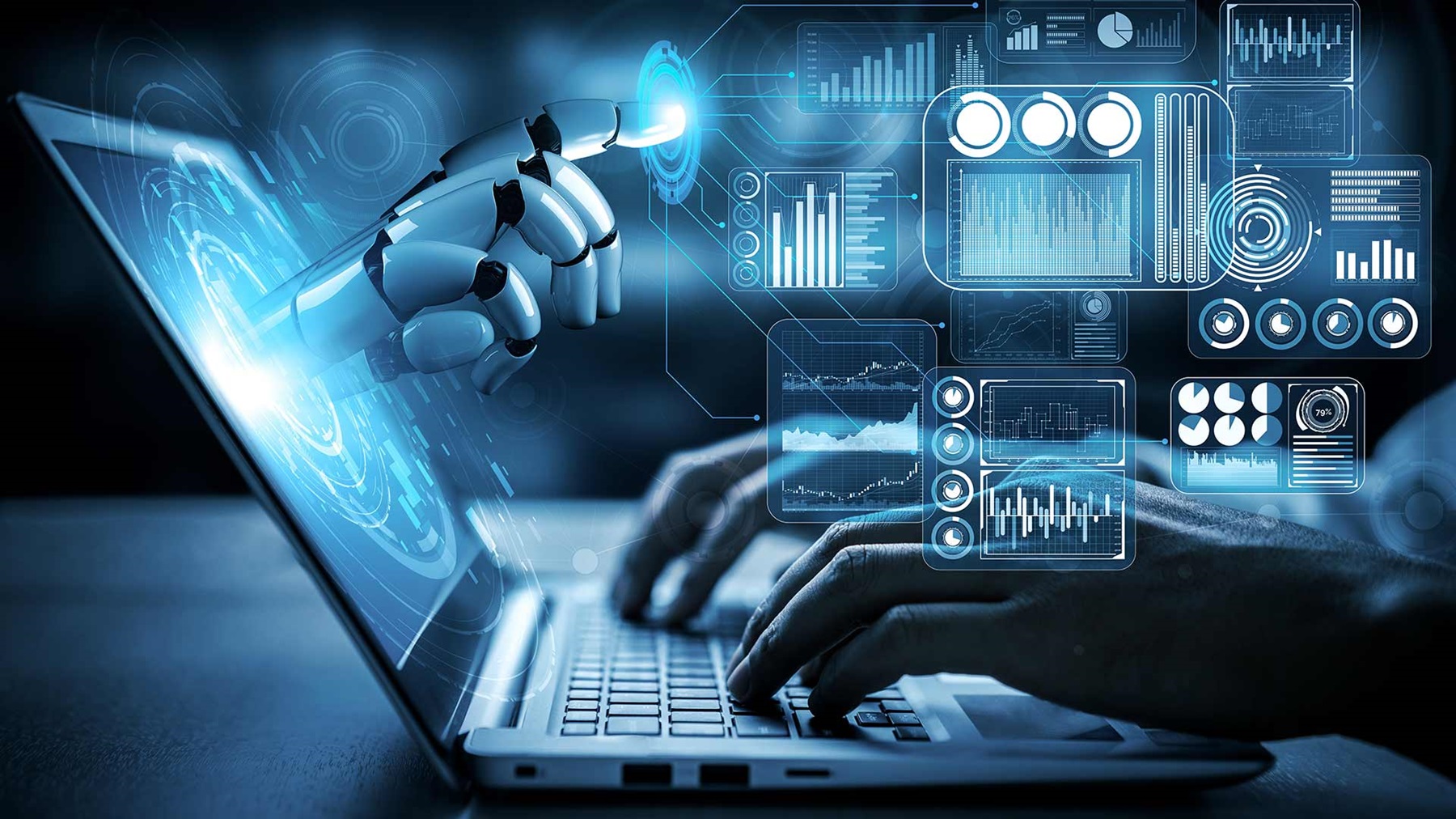
Fans of the Swedish pop group ABBA can now see them in concert and experience them in their heyday of the 1970s, as digital versions or ‘Abba-tars’. This virtual concert in London is part of a growing trend of replacing humans with artificial intelligence (AI), and now Korean pop music – or K-Pop – is doing something similar. The group Eternity recently released ‘I’m real’, an ironically named song that featured its 11 members. And just like the ‘Abba-tars’, they aren’t real or human – they are virtual characters that have been created with AI.
If our basic entertainment is moving in this direction, what about the rest of the business world? Will we need humans in the future? What kind of decision-making will we leave to AI? And if we do that, what could possibly go wrong? Such are the questions of science fiction fandom, but for treasurers – and their corporations – the answers are fortunately a lot more mundane.
The use of AI and machine learning (ML) is consistently mentioned as a major trend by the treasurers that Treasury Today regularly speaks to. Dr Andreas Bohn, Partner at McKinsey and expert in treasury management, risk management and capital markets, explains that the main uses of AI by corporate treasury are for cash flow forecasting, optimising hedging decisions, forecasting the parameters of the markets and improving data quality.
Are these applications risky in anyway? Could the machines be running away with their own learning and make erroneous decisions? “There is always a risk of getting forecasts wrong,” says Bohn. He comments that the issues usually relate to the data that the applications draw on. The data quality might not be appropriate, someone might have manually input it, and there might be errors. “The algorithms need to protect themselves against data errors,” says Bohn. He adds that applying the human-in-the-loop concept, where AI and ML is developed with the involvement of humans, is important to ensuring that the technology is developed and used in a way where risk appetite, business needs and specifications are aligned.
As yet, it does not seem that treasurers are over-relying on these tools and heading for a science fiction scenario. “If people make mistakes it is more on the reliance of the status quo and not being able to imagine scenarios that would be outside the usual parameters,” Bohn comments. For example, during the energy crisis, some were unable to even conceive that energy firms would go bankrupt or that energy prices would skyrocket. “When these algorithms are implemented it is still more in the testing phase than anything else. What I have perceived is that they are used on the treasury side as an additional tool, which is a backup and accompanies regular activities,” adds Bohn.
When it comes to the riskier aspects of AI, Ben Rapp, Founder and Principal at Securys, a specialist data protection and privacy consultancy, comments that treasury’s use of the tools is unlikely to be a concern. The ethical dilemmas of AI, which are becoming a hot topic for businesses and regulators alike, are more likely to be a challenge for financial institutions than corporate treasury, he notes.
More broadly, businesses are relying on AI and ML for strategic decisions. Andrej Zwitter, Professor of Governance and Innovation at the Netherlands’ University of Groningen, says there are some very important considerations when implementing AI as a strategic decision-making framework. With traditional analytics and business intelligence, he explains, it is clear what is going in, in terms of the data, the process that will be applied and the different analytic techniques that will be used – and the outcome is transparent. “With automated decision making there is data scraping, and the quality of the data can often not be assured.
“Also, the process of AI is using convoluted statistics and complicated mathematics is being applied. Because it is so complicated it is not possible to understand what the decision is based on – it is a black box.” Zwitter adds, “There is no way to second guess the advice it gives.”
There are numerous examples of organisations making decisions based on biased data. Zwitter points to an example of the Correctional Offender Management Profiling for Alternative Sanctions (COMPAS) tool, which was used by courts in the United States to predict the recidivism rates of offenders. However, it was later shown that this artificial intelligence had an inherent racial bias because it was based on population data from US jails, which had a higher proportion of African Americans. Also, because the software was proprietary, the judges were unable to look into how the judgements about offenders were made, explains Zwitter.
Rapp at Securys also raises this issue of the training of data, and gives the example of Amazon, which had to discontinue a recruiting tool it introduced in 2014 because its predictions of what an ideal software developer looked like used biased data. The machine was effectively learning – and then predicting – that a ‘good’ candidate was white and male. Rapp explains that once the tool is on this path, it becomes almost impossible to correct it, as you’d have to balance out this bias with an almost equal amount of data – on female software developers, for example – that doesn’t exist.
Meredith Broussard, an Associate Professor at New York University and author of More Than a Glitch: Confronting Race, Gender and Ability Bias in Tech says, “There is a perception that using data to make decisions is more objective and more unbiased – that is not true. There is no such thing as an unbiased dataset.” She gives the example of financial institutions building a solution that helps decide on who gets approved for home loans; that dataset would use who got mortgages in the past. “What social scientists know about lending is there is a history of discrimination of who has gotten home loans in the past and that past discrimination is reflected in the data,” Broussard explains. She points to The MarkUp’s investigation into racial disparities in mortgage applications and argues that all businesses should be doing algorithmic accountability reporting to test for bias in the data.
Rapp comments that financial institutions – and other businesses – will have to care about these issues because the European Union will soon be regulating the use of AI, which will cover issues such as transparency and the right of recourse. “There are broad questions about the power and the asymmetry of the relationship with the borrower,” he says. “These regulations are going to hugely increase the scrutiny of those systems and institutions are going to be subject to much bigger fines if they cannot show they are being fair.”
AI will be categorised in tiers according to its level of risk – ranging from unacceptable and high risk to low or negligible risk – and if businesses fall foul of the regulations they could potentially be fined up to 6% of global turnover.
Rapp outlines some areas that may be challenging for financial institutions. Anti-money laundering transaction screening and the profiling of customers can have a deleterious impact on customers, particularly when there are false positives and a ‘good’ customer is blocked out of their account based on an automated decision.
Also, there are issues with using biometrics to allow access to a bank account. This could be done through key stroke patterns, or facial features. But if a customer has hurt their finger and can’t type properly, or has a black eye – or had recent plastic surgery, for example – they will be locked out of their account even though they are a genuine customer.
When asked if there is an unthinking over-reliance on these tools, Rapp says that the people working with them and implementing them are aware of the ethical issues involved. When it comes to the general public, however, especially if they have watched a lot of science fiction films – they are more likely to think that AI is more intelligent and powerful than it actually is.
Zwitter agrees. “These tools are referred to as smart and intelligent, but these words are metaphors – they have no real meaning. These tools are not intelligent or smart. They are algorithms, essentially mathematical formulas that are based on data and certain rules – there is no intelligence or smartness,” he says.
Rapp agrees and says, “They are tools that are trained to do specific tasks. They can be remarkably good at that and seeming smart.” In fact, he adds, sometimes when people think AI is being used to answer questions, it is actually a human in a call centre typing a response. And on a similar topic, Broussard recommends Behind the Screen: Content Moderation in the Shadows of Social Media, a book that examines the use of human moderators – and not algorithms – in evaluating posts on mainstream social media platforms.
“AI is great for a variety of applications” particularly automating boring tasks, says Broussard. “However, people run into trouble because they imagine AI can do more than it actually can. When people are imagining that AI is going to be sentient and replace humans they have to keep in mind of what it is good at and not good at – and balance the expectations. Even the name artificial intelligence and ML is misleading – it suggests there is a brain in the computer,” she says.
It is easy to imagine that AI will lead us down a path where the machines have taught themselves enough, will take over from humans and the technology will be uncontrollable – the point of singularity. Is this a real danger that we need to be concerned about? Is humanity doomed? On the question of whether we will reach singularity, Zwitter says, “It is impressive what AI is able to do, but we are giving it too much credit – it is literally just zeroes and ones. There is no intentionality or agency; there is no such thing as intelligence with an opinion unless we point it somewhere. There is no reason to believe there will be a point in time where humans lose control over AI – unless we give it all the control and we outsource our moral agency to these tools,” says Zwitter.
Broussard agrees that AI doesn’t threaten the future of humanity. “One thing I would be concerned about though is autonomous weapons and the commercial availability of food delivery robots – and people doing things like attaching guns to them,” says Broussard. She also points to the example of police in San Francisco proposing to allow police robots to kill suspects, which Broussard describes as “a terrible idea”. After a similar response from the public, the proposal was reversed in early December 2022.
For corporates and their treasuries, it seems there is no need to worry about the machines taking over. The prospects of a science fiction dystopian nightmare are low, and when they see their favourite musicians they will most likely be human. For now.