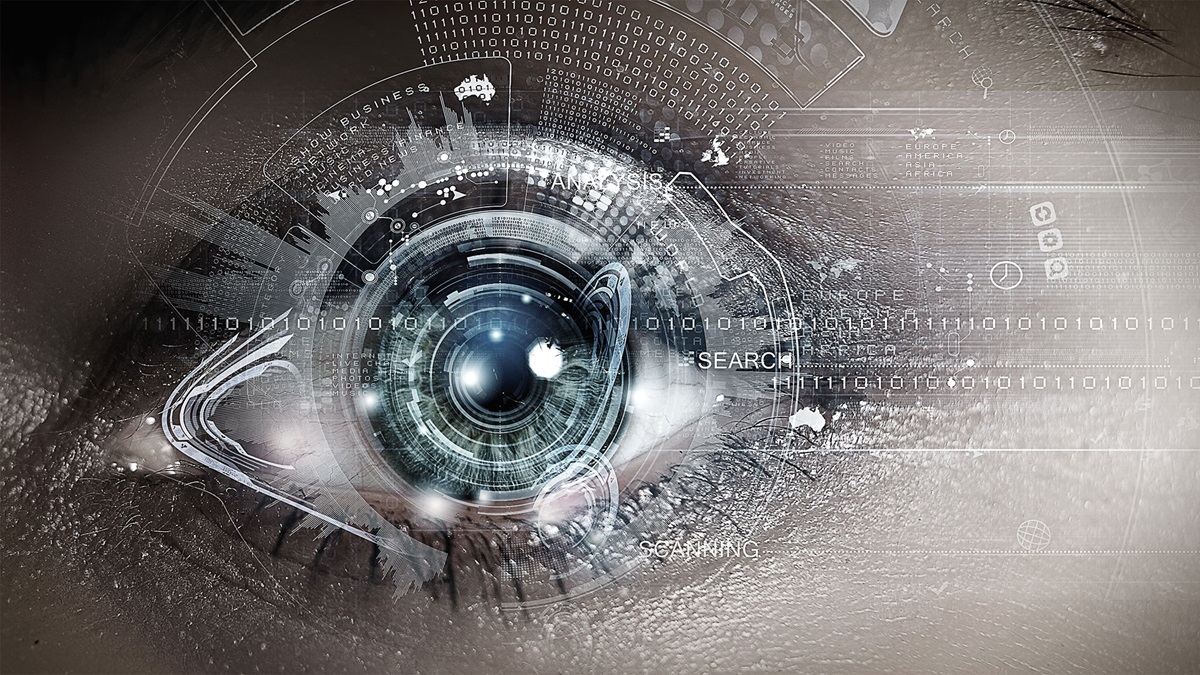
We look at how AI can be used to drive greater efficiency and accuracy in fraud detection and sanctions compliance and probe the issues that its adoption may present.
The world is awash with data. When it comes to matters of compliance monitoring, especially around sanctions screening and fraud detection, the traditional ‘human operative’ approach to analysis looks increasingly difficult and yet is still very much in evidence.
This means that in a progressively real-time world, businesses and banks face a direct and indirect threat from real-time crime. The answer to the problem of too much data, and too many actors with bad intent, in this environment is to fight fire with fire, deploying yet more technology.
Certainly, the world’s major banks think so. A global study of 400 bank executives conducted in March 2018 by The Economist found that 71% of executives are focusing their digital investments on cyber-security. And for good reason. According to a Javelin study, major banks lost US$16.8bn to cybercriminals in 2017. This figure, notes Forbes’ analysis of the study, includes regulatory fines, litigation, additional cyber-security following the breach, the need to respond to negative media coverage, identity theft protection and credit monitoring services to customers affected by breach and lost business due to reputational damage.
It is interesting to note that The Economist’s study also revealed that around 30% of respondents saw AI platforms as a key part of their digital investment. But then the capacity for AI to sift through, analyse and report on vast and disparate data sources goes hand-in-hand with the rising threat of financial crime.
Just an algorithm
A simple definition of AI can reduce it to an algorithm or a set of rules that are followed to form an outcome. It may then be seen as having a ‘narrow’ purpose, where it is used for a specific use case (such as online shopping assistants), or as a ‘general’ solution where it exhibits so-called ‘deep learning’ capabilities more akin to humans (such as the Deep Blue and Watson supercomputers).
AI in a fraud and compliance application is narrow in scope, albeit both a simple data-crunching exercise across a vast data pool, and a fairly complex, long-term pattern-recognition system. At some stage it could become part of a general ‘neural network’, capable of predicting the behaviours of and making decisions about multiple actors within the broad-ranging financial sector.
For now, it is only feasible to deploy AI to detect changes in activity where perhaps a new beneficiary is being paid or an account number has changed on a regular payment. But here it can flag anomalies (sometimes referred to as outliers) over a huge set of transactions and an extended period, that no human could ever match. These unusual values could be a single data point or a general trend or behaviour observed in that data set.
For Emma Loftus, Global Head of Payments, J.P. Morgan, AI has an increasingly important role to play in financial services. And for good reason. “With wire business, while it is easy to use standard rules to filter everything out that does not conform, it is a blunt instrument. AI allows more nuanced filtering,” she explains.
Looking across 12 months of payments activity, it may be possible for AI to see that what at first appears to be abnormal, is in fact a regular but low frequency transaction that exceeds the norm. This, Loftus says, enables the bank to give the client a lot more context on why it has called out a specific instruction, or to process it unhindered.
Status quo
In terms of its ‘traditional’ general cyber defence, a major banking institution will commonly deploy at least a three-tier approach, says Loftus. In this context, it has to protect its perimeter, erecting internal levels of control around money movement and augmenting this with a strong education programme with its clients to encourage digital security best practice.
The second layer, where controls around payments operations are supported, sees a number of systems in place. The bank has to detect, for example, when client computing and credentials have been compromised, ensuring any access attempts that do not conform to expectations can be managed with a high degree of precision.
Where uncharacteristic account activity is spotted, the bank’s proactive response to such findings has, for example, seen it close down unused SWIFT Relationship Management Applications (RMAs) with institutions. It has also enforced its own payment access limitations where there is no longer a reason for an individual to have that permission.
Loftus adds that clients are encouraged to review their own auto-procedures and authorised transaction initiators and signatories. The bank supports this with internal controls around, for instance, transaction limits where bank contact is mandated to seek verification beyond a certain transaction level or where a new beneficiary is detected. Indeed, as she notes, “first-time beneficiaries are often where risk is heightened and a simple call-back would stop a lot of fraud”.
Artificial assistant
So far so good, but a reminder that banks lost US$16.8bn to cyber-criminals in 2017 brings us to the deployment of new weapons in the war against cyber. Here, AI has a role to play in protecting banks and their clients.
With the move gathering pace (certainly in the consumer space) towards real-time payments and the credit ‘push’ model, Loftus says the idea that fraudsters can navigate rapidly across the consumer space, using ‘mule’ accounts spanning multiple institutions, is driving greater interest in AI. Indeed, she believes that AI is becoming “necessary” to properly secure clients’ money movements because only this type of technology is able to effectively and efficiently collect, collate and analyse data across vast numbers of remitters and beneficiaries.
In action
Basic AI is already being used to enhance the efficiency and seamlessness of processes in fraud detection and sanctions compliance, says Kristian Luoma, Head of OP Lab, OP Financial Group (one of the largest financial companies in Finland, consisting of 156 cooperative banks). For him, AI is only just at the stage of “intelligent assistant”, augmenting the work of its human counterparts. “It may be a while, if ever, before an algorithm is trusted fully in this context,” he comments.
However, he recognises that the advance of new digital channels and the exponential growth of data is driving deeper interest in AI. As Loftus says, it has to. Indeed, Luoma believes that it is the greater degree of accuracy promised by AI, and the understanding that machines are better at executing certain tasks – such as the detection of long-term patterns of behaviour – that will encourage its uptake.
The reasons for not adopting AI as a superior mitigation of financial crime are beginning to melt away. But there are pure commercial reasons for its uptake too, says Luoma. “We can already see that the entire finance landscape is being reshaped by competitors that do not necessarily share the physical challenges of traditional banks,” he comments. “Almost by definition, offering a digital-only interface potentially gives these players the upper-hand on cost structure. As incumbents, unless we are ready to use technology to help drive down costs, there is a real possibility that we won’t be as competitive in terms of our pricing to customers.”
Advancing AI
Financial institutions have been increasingly incorporating unstructured data from external sources, such as news feeds and social media, into their financial crime and compliance investigations, says Leonardo Orlando, an executive in Accenture’s Finance and Risk practice. But this approach, he notes, has always proven costly in terms of resources. Some institutions have therefore sought to embed into their systems intelligent web crawlers, capable of automatically retrieving such data.
But as tackling financial crime takes on a new urgency, banks are looking for ever more accurate and efficient solutions. Orlando says some banks are beginning to deploy more advanced AI, in the form of machine learning technology. The ability to analyse existing transactions to detect outliers, and extrapolate patterns to form a structured future view, means these systems offer a clear advantage over basic AI.
These systems can begin to make self-directed decisions as the algorithm learns the mapping functions, from input to output. Accuracy improves as more and more relevant variables are embedded in the rules, even when using data from unstructured sources such as social media. This ‘supervised’ form of AI, where humans ‘train’ the model step-by-step, is sometimes referred to as intelligent automation.
At the cutting edge of AI in this context is network analytics. This, explains Orlando, is designed to seek out all the knowable connections of a business or individual. Where one otherwise above-board organisation seems to be connecting with another that, although itself clean, has some questionable associates further down the line, investigation may be triggered. This might especially be the case if the first organisation is generating unexplained transactions within its own network. This level of depth and breadth of analysis would not be possible using traditional models. Some may find this intrusive.
No takeover
Whilst Luoma is not predicting a machine takeover, as a technologist, he is not prepared to say there are elements of cyber that AI can never learn. That said, he suggests that few if any organisations are anywhere close to letting it “fly solo”. Even if in most cases AI can produce a better result than a human, he accepts that there may be circumstances where human intervention is necessary; humans having a far broader set of experiences and knowledge upon which to draw.
The unending race between those engaged in malicious acts, and those seeking to detect and prevent is a case in point. AI can learn but currently an algorithm is “only as skilled as its training data allows it to be”, Luoma notes. It is not yet configurable to recognise new types of fraud; it can only see anomalous effects after the fact. For this reason, for now at least, he argues that human involvement is essential.
In fact, despite the adaptive and resourceful nature of those who seek to commit financial crime, banks and businesses are not scrambling to implement AI out of fear for what may happen at the hands of criminals. Instead, says Luoma, organisations that are adopting it are doing so as a measured and rational response to ‘normal’ business challenges. In short, some are already seeing the commercial opportunities.
“I don’t see fear of the existence of this new technology, nor do I see the pledging of blind allegiance to it,” he says. “From our own perspective, if there is a tool to help mitigate risk and help increase the value that we are able to produce for our customers, then that is an opportunity we should take. It is an opportunity to be more efficient with less resources through the use of humans and AI.”
Recognised issues
It’s a given that where the AI opportunity is taken, the biggest issue will always be the quality of the data being analysed. However, another concern with AI is calibration of an algorithm to create an appropriate feedback loop. Traditional rules-based systems lack the nuanced capabilities of AI, but poorly calibrated AI is at best unhelpful.
An algorithm must be calibrated to avoid too many false positives or false negatives, Loftus explains. Here, transactions are unnecessarily stopped or allowed because the algorithm is either over- or under-sensitive. At best it annoys clients when their transactions are stopped unnecessarily. At worst it allows criminal activity to pass undetected. It is a difficult balance to achieve but one that nonetheless must be tackled.
Moreover, an algorithm that incorporates bias – usually unintended but incorporated in its decision processes as a result of historical or cultural norms – can detract, to similar effect, from an otherwise successful system.
Treasurers get ready
Fraud detection, anti-money laundering, and sanctions and watchlists screening form an essential part of every bank’s ongoing processes. These actions have not traditionally been part of the treasury remit. However, suggests Orlando, treasury has a unique view over every transaction of the business. As such it is in a position, even before any transaction has been settled with a counterparty, to assess if there is any fraud or compliance risk.
AI technology is now being deployed by some TMS providers, Loftus notes, helping treasury departments become a “first line of defence” in detecting anomalous internal activity. But, says Orlando, it may be time to go further.
Treasurers could, for example, use AI to better understand multiple counterparties in hitherto unseen depth. Knowing where a counterparty is winning or losing contracts, its investment in strategic activities such as M&A or R&D, current and historical investor sentiment, and even ongoing legal cases, can paint a picture of an organisation in ascent or decline.
As a risk management tool, he argues, this surely is valuable data. As a means of improving forecasting accuracy, where customer payments can be predicted far in advance, he suggests that it has a ready-made strong business case for treasuries seeking to optimise liquidity and capital.
Time to jump?
Where AI is being adopted, for it to gain acceptance and trust, the business needs to be confident that its algorithms are working as intended. Orlando advises initially limiting the scope of what is trying to be achieved. “Start small and work on elements that can be monitored and controlled. Get comfortable with it first. Understand how it works and where the value exists. From there you can try to expand the scope in an organic and structured way.”
Regardless of how AI is approached, Orlando insists that planning is essential and that it is therefore vital to “connect the dots between the right data, technology and solution”. Jumping to the conclusion that AI is the answer to every problem, he warns, is a recipe for failure.
Of course, organisations that are exposed to the increasing risk of financial crime, are in no way obliged to adopt AI just to meet regulatory requirements. On the other side, the enormous potential of a well-balanced AI solution is something that should be considered, and treasurers have many reasons to be at the cutting edge.